One of the coolest features of Aampe is that it lets you inject your product catalog into push notifications. So if you're an e-commerce app, you can quickly write messages about specific categories, and Aampe will deliver those according to your user preferences. Your hiking lovers will receive a push notification about the latest equipment, and your fashionista users will receive a push about the hottest brand in town.
Push notifications are a fantastic discovery engine. They deliver value to your users without all the strenuous scrolling. Less scrolling: happier users!
But why stop at the level of the category when you can make your push notifications even richer and help your users directly discover specific items in your catalog?
What Aampe does is combine the user preferences that it learns via messaging (Sami likes casual wear) with a recommender system based on the consumption history of all other users. The result is a push notification that can look like this:
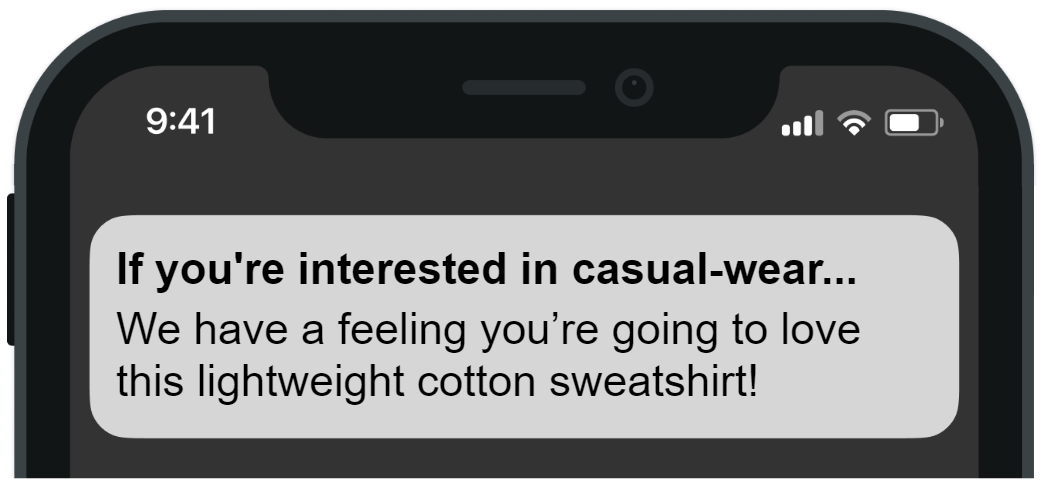
Under the hood
The following will be a short review of our design decisions and algorithm choices in building a recommendations engine that is generalizable across customers and scalable for large catalogs. Skip to the "How does this play out in real life?" section for a demonstration of behavior if you're not very interested.
Design considerations
Coming to design a recommendation engine, we faced with the following constraints:
- It should be generalizable to all of Aampe's customers
- It should support large item catalogs (>10M)
- It should support large user bases (>50M)
- It cannot assume there is any item rating data
- It should cater to the long-tail
- It cannot afford a long cold-start and should be able to start recommending after the first consumption event and
- It should be cost-efficient
Going through the above constraints, one can see that collaborative filtering techniques, on which many recommendation engines are based, are not ideal. They would have suffered from the data sparsity, cost a fortune in matrix factorization, and most probably failed to deliver valuable recommendations following only a short consumption history.
Content-based filtering recommendation techniques are a good alternative, but those wouldn't easily generalize across the Aampe customer base. Different customers come with varying levels of catalog metadata and we didn't want to build an algorithm that only works for a subset of customers (or would require a huge amount of data organization work).
Instead, we built a recommendation engine that is based on item consumption sequences. The base assumption of this technique is that if a user consumes item A and then item B, a different user consuming item A will also be interested in item B.
Let's take a look at this simplified item consumption graph of user blue and user red for an illustration of this base assumption:
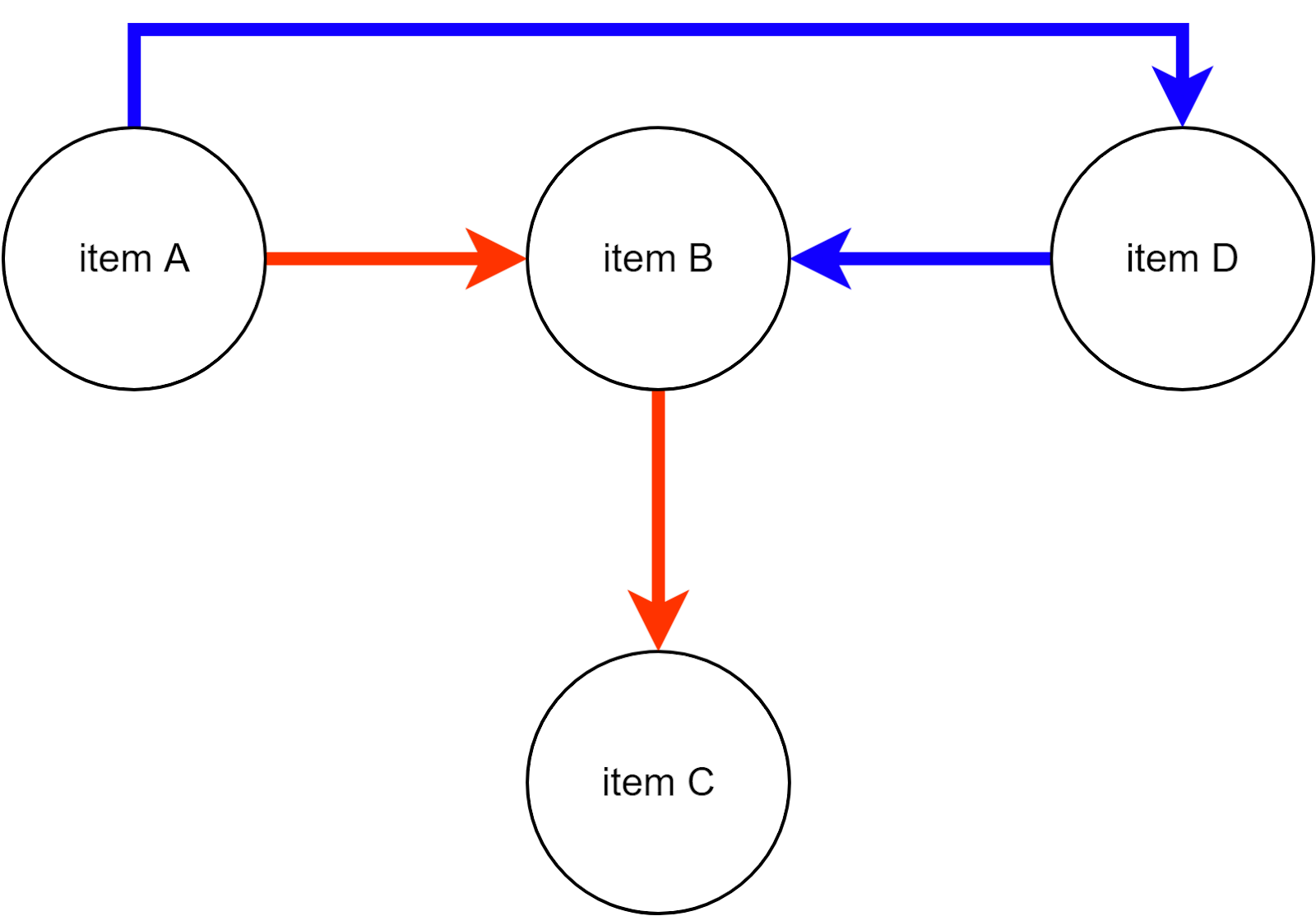
First, the facts:
- User Red consumed items A, B, and C; and
- User Blue consumed items A, D, and B
Now for the illustration of the base assumption: because user blue consumed item D after item A, and user red consumed item A, there is some likelihood that user red would be interested in item D.
Algorithm
- Maintain two tables for all users
- Consumption history: a record for all item consumption events with timestamp and item_id
- Messaging history: a record for all items ever messages about (so as not to recommend the same item over and over again)
- Build an item-to-item transition matrix from the consumption history matrix that captures the likelihood of consuming item B having consumed item A. The likelihood score is a function of how many users make the consumption sequence, the time between the two consumption events, and the type of consumption event (add_to_cart, purchase, etc.).
- Generate a recommendation for user A:
- Joining their consumption history with the first item in the transition matrix
- Aggregating over the second item
- Sort the aggregated scores in a descending order
- Pick the item with the higher score that does not appear in the messaging history table
Evaluation
If we revisit the list of constraints, we can see that this algorithm is generalizable because it doesn't need rich catalog metadata or item ratings. It also involves relatively straightforward computations and therefore scales easily to millions of catalog items and 10s of millions of users cost-efficiently. This algorithm can also recommend items starting the first consumption event (no cold start problem, at least for active users). But what's really interesting is that catering for the long tail comes built-in when using consumption sequence recommendations. A niche category user will immediately access the transition matrix in that category's rows and receive recommendations about their niche items of interest without any algorithmic juggling.
How does this play out in real life?
User journey
Here's the consumption activity of a real user looking at trekking equipment in an e-commerce app to give you a glimpse of how the system behaves. This user browses packs, tents, poles, an umbrella, and a sleeping bag. They also add the tent and the sleeping bag to the cart.
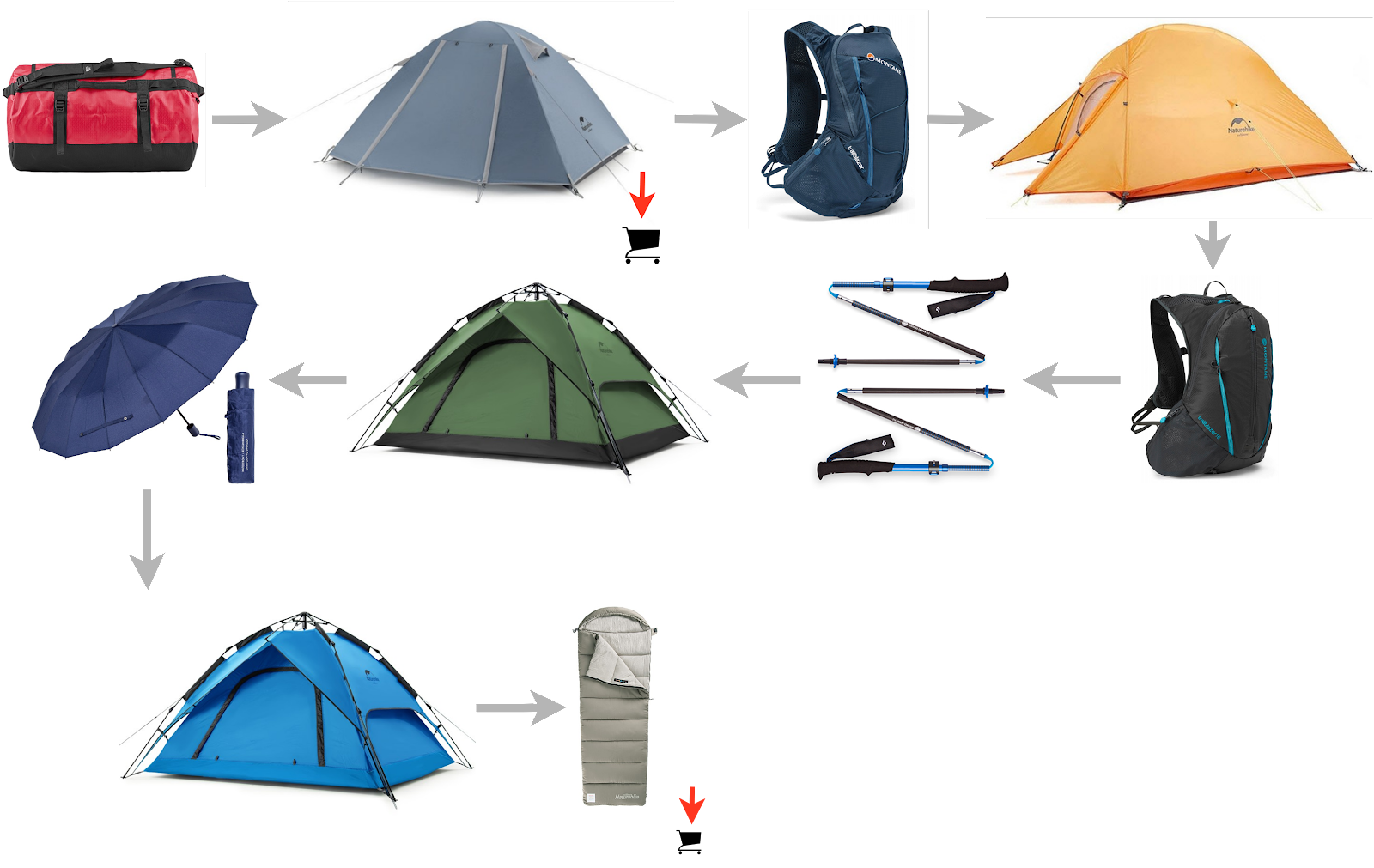
Recommendation
Sometime later, when that user qualified for a recommendation push notification, they received the following message:
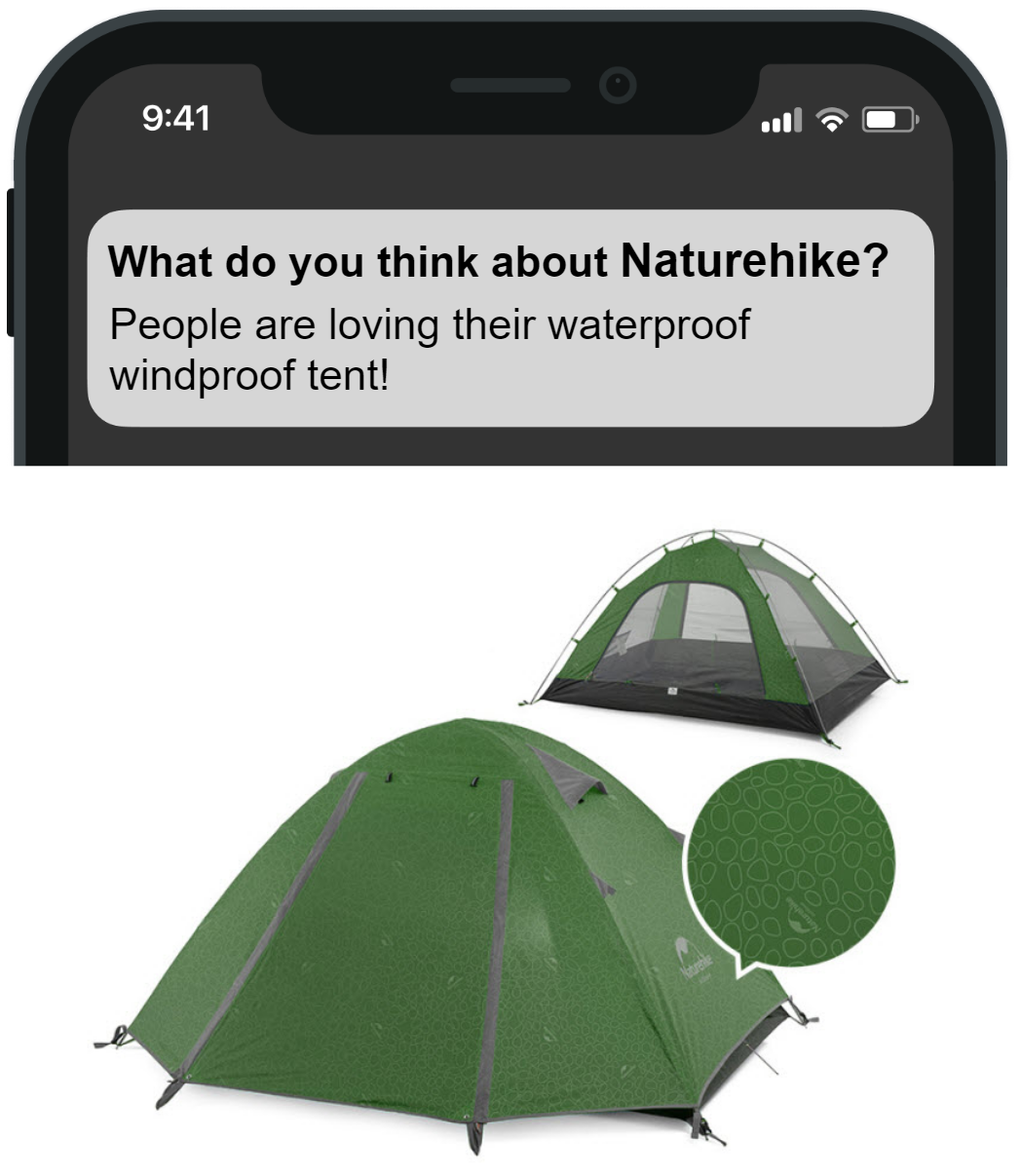
Contenders
We can also introspect the algorithm by looking at the recommendation matrix generated in step 3 above and find the top 5 items (following the one that was recommended) for that specific user and their likelihood scores.

Remember, those potential recommendations are all based on what other users consumed following a consumption history similar to the user looking for hiking equipment we're looking at here.
Are you interested in injecting your product catalog into your app's notifications? Drop us a line via hello [at] aampe.com!
Do you want to help us build systems like this one? Ping us via talent [at] aampe.com; we're always keen to get to know you!