As an average of 70.2% of eCommerce carts are abandoned—meaning, out of every ten people who begin the checkout process, seven of them don’t complete it—abandoned cart messaging has become a crucial capability in the eCommerce industry.
(Forrester estimates that abandoned carts cost the eCommerce industry $18 billion in lost sales every year, so spending time developing an abandoned cart messaging strategy is undoubtedly justified.)
...but is abandoned cart messaging the best way to capture and increase conversions?
Abandoned Cart Messaging Statistics
We analyzed over 2 million messages sent by a popular eCommerce app to over a million users in three different countries, and here's what we found:
Abandoned Cart notifications were 20 times more effective than general marketing notifications at driving clicks and additional add-to-cart events, 24 times more effective at driving general engagement, and 25 times more effective at driving check-out events.
These are impressive numbers, and when you think about it, they totally make sense because you’re not just messaging any potential customer—you’re messaging a high-intent customer who has gone so far as to add a product to their cart.
The main drawback of abandoned cart messaging
With stats like these, why wouldn’t we send abandoned cart messages to everyone?!
Well, it’s obvious, isn’t it?
This is the main limitation of abandoned cart messaging: You can only send abandoned cart messages to users who have actually abandoned a cart. In our study, this was only 4.6% of users. Abandoned cart messaging was much more efficient at driving incremental purchases, but it only drove 526 additional purchases during this time period. The number of purchases attributed to all other notifications was over 4.8k.
To be clear, this isn’t a reason to stop sending abandoned cart notifications. This is a reason to find better methods to help increase purchase success rates from a larger percentage of your population.
First, let’s look at how to make abandoned cart messaging more effective and then we’ll look at an even better method.
How to construct an effective abandoned cart messaging strategy
When it comes to getting users to complete their purchases, your messaging channel and timing have the biggest impact on success.
The most effective abandoned cart messaging channels
Abandoned cart emails have long been the gold standard, but that’s quickly changing.
Email was historically prominent, but now, with smartphones accounting for 72% of all retail site visits and 61% of online shopping orders in the first quarter of 2023, retailers need to adopt a true omnichannel strategy.
According to BusinessofApps, push notifications:
- Have a 10x higher open rate and a 14x higher open rate than emails
- Have a 3x higher opt-in rate vs. emails.
- Get near-instantaneous reactions vs. an average 6.5-hour reaction timing for email.
- Oh, and they don’t end up in the Spam or Promotions folder.
…but despite these impressive statistics, push notifications still might not be the best method to reach your customers.
For example, SMS messages can get an open rate 4.5 times higher than push notifications, but they often come with more baggage. While many customers are used to getting push notifications from eCommerce apps, some view SMS as a more private channel, and they see receiving SMS notifications as intrusive.
Some customers prefer SMS notifications, while others prefer push notifications or even emails. The most important thing is to send your customer the communication on the channel(s) they prefer and at the timing that’s useful to them.
Should you send abandoned cart SMS or push notifications? Check out our comparison here.
The most effective abandoned cart timing
What’s the best timing for sending abandoned cart messaging? We have all the data here.
Product Recommendation Messaging: A more effective way to drive eCommerce conversions
Now that we’ve discussed abandoned cart messages, let’s look at a method that is less efficient but much more effective.
What are product recommendation notifications?
Product recommendation notifications are similar to the standard product recommendations you likely offer on your site or app, but instead of waiting for your customer to come to your site or app or perform a search, these recommendations are proactively sent to your users via one of their preferred notification channels (e.g., SMS, In-App, mobile/web push notifications, WhatsApp, etc.).

Product recommendation notifications are so effective because they combine the effectiveness of proactive messaging, mentioned above, with the incredible effectiveness of product recommendations in general:
- Salesforce found that, while item recommendations make up only 7% of eCommerce traffic, they account for 24% of orders and 26% of revenue.
- They also found that consumers who interact with product recommendations are 4.5x more likely to add items to their cart and complete a purchase, and 52% of orders from customers who clicked on product recommendations include a recommended product.
- 49% of consumers said they have purchased a product they didn’t initially plan on purchasing after receiving a personalized recommendation.
- Finally, 54% of retailers claimed that product recommendations acted as the key driver of AOV (average order value) in customer purchases, and product recommendations accounted for up to 31% of eCommerce revenues.
Even if a shopper didn’t buy anything, shoppers who clicked on product recommendations were found to be 20% more likely to return to the retailer later.
The effectiveness of product recommendation notifications
Let’s be clear: product recommendation notifications are NOT as efficient as abandoned carts, but they have a distinct advantage that makes them more effective: they can be sent to anyone.
A customer does not need to abandon a cart to be eligible for a product recommendation message. They just have to exist.
So, if we were to extend the same analysis we did for abandoned carts above, it would look like this:
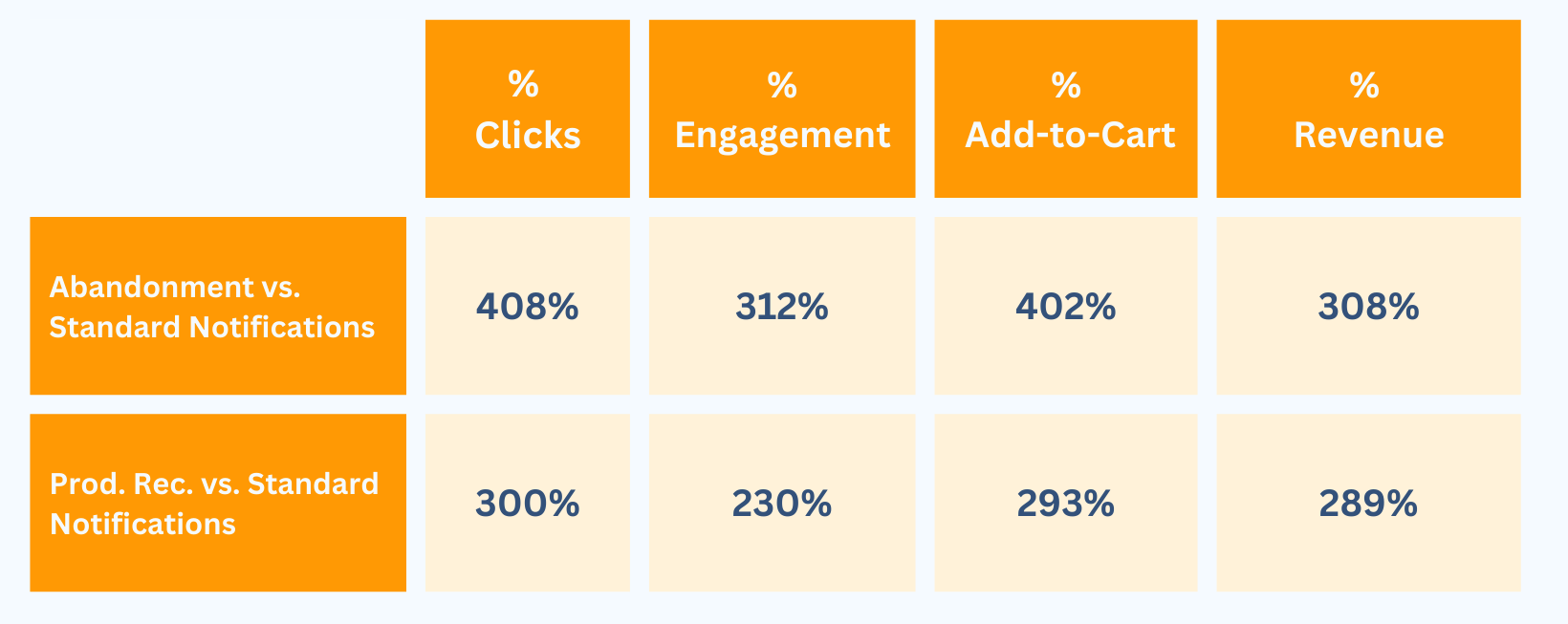
In other words, while Abandoned Cart messaging was 308% more effective at driving purchases (“Revenue events”) compared to general or “standard” notifications, Product recommendation notifications were only 10% less effective at driving conversions than abandoned cart messages.
They were still 289% more effective at driving conversions compared to standard marketing messages.
If we extrapolate these results across messaging volume, we can really see the impact:
While abandoned cart messages drove 526 additional conversions out of 86,948 messages sent, this app was able to send almost 6x the volume of product recommendation messages, driving just under 3,000 purchase events—almost 1,000 more purchase events than the 1.2 million general notifications sent over this same time period.
This proves that efficiency doesn’t always equal effectiveness.
But, of course, you have to be sure you’re sending the right type of recommendations.
The two most common types of product recommendation engines
Most product recommender engines boil down to two main types:
The most common recommender type is a collaborative filtering recommender. Collaborative filtering looks at what your user bought and what other, similar, people have bought and recommends items bought by people who have similar buying patterns to the original user. A collaborative filtering recommender ends up sending your users lots of very similar recommendations (for example, after a user buys socks they may keep getting recommendations for only the most popular shoes and underwear).
The second most common type is a content recommender. This recommender type looks at an item’s attributes and tries to predict which attributes your particular user likes. With a content recommender, if a user buys yellow socks, they may get recommendations for yellow shoes and shirts next. Content recommenders require a huge amount of infrastructure such as data and engineering, which translates to time and money.
Both of these types are computationally expensive, as they crunch very large datasets in a way that takes a lot of computer power.
The challenges with most common recommender engines
As mentioned above, most approaches to recommender engines are computationally expensive which makes them physically expensive, but aside from cost, there are several other drawbacks:
Recommender systems can quickly become popularity contests (showing only the most viewed items), and they don’t take into account actual buying logic — buying socks means a user is probably less likely to buy socks, and buying something yellow doesn’t necessarily mean your user likes tons of yellow things — buying typically happens in a sequence, and neither approach takes sequence into account.
Both approaches are also labor-intensive to set up and require constant maintenance and upkeep to stay relevant.
How to overcome the challenges with typical product recommender engines
Here’s how our approach to product recommender systems is different.
- We look at all interactions with items for each user. We don’t just look at purchases. We consider every interaction including views, shares, adds to wishlists, add to carts, and purchases. Sure, we weigh things towards the bottom of the funnel heavier, but we use all relevant events in our modeling.
- We use all of the weighted histories we collect to build a network of interactions (e.g. “User viewed item X then added item X to the cart then viewed item Y then bought item X, etc.) Items get connected by their sequences in individual histories rather than by user similarity. We use this network to construct a “similarity matrix” that tells us, given the interaction with any particular item, how likely each other item is to be interacted with.
- We also model the purchase probability for each item (So, given that a user looks at an item, how likely are they to purchase something — even if it’s not the same item they viewed today — the next day?)
- After doing that groundwork, we then take an individual user history and plug that history into the item similarity matrix. Combining all of those connections ends up giving us a list of items, scored by how good of a “next choice” they are and how high of a “purchase probability” they carry, which is what we recommend.
There are a few distinct benefits to this approach:
- We don’t have to actually know any attributes about the item being recommended, which makes our system incredibly adaptable and scalable to a variety of industries and catalogs.
- We fix the cold-start problem.
The cold-start problem is a common issue with recommender systems — What do you recommend when a user has no purchase history?
This issue stops most recommenders dead in their tracks. For us, we just go straight to our purchase probability score.
Our recommender system is typically the opposite of a popularity contest.
Many of the most popular items in our customers inventory don’t actually have a high conversion probability. There’s a big difference between what people like to look at and what they actually buy.
How can you send personalized product recommendations in your eCommerce app?
Integrating personalized product recommendations into your eCommerce app is surprisingly easy.
Here are the steps:
First, directly integrate your CMS into Aampe
There’s no need to enter products by hand or even do much special formatting. We just need to know the products that are available in your inventory.
We can also ingest information like your current deals and stock levels and combine it with our logic to send abandoned cart messaging or messages that offer discounts on items recently viewed but not purchased.
Secondly, type messages just as you normally would

Third, add a liquid placeholder for your product recommendation
Just click on the little shopping cart icon and then your product name field from your CMS:
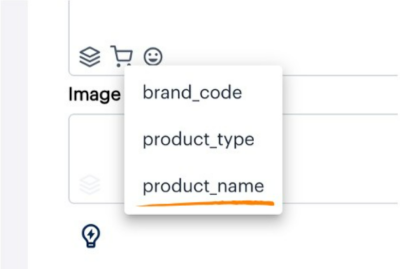
Compose the rest of your message, including a Greeting, CTA, incentive and other best practices
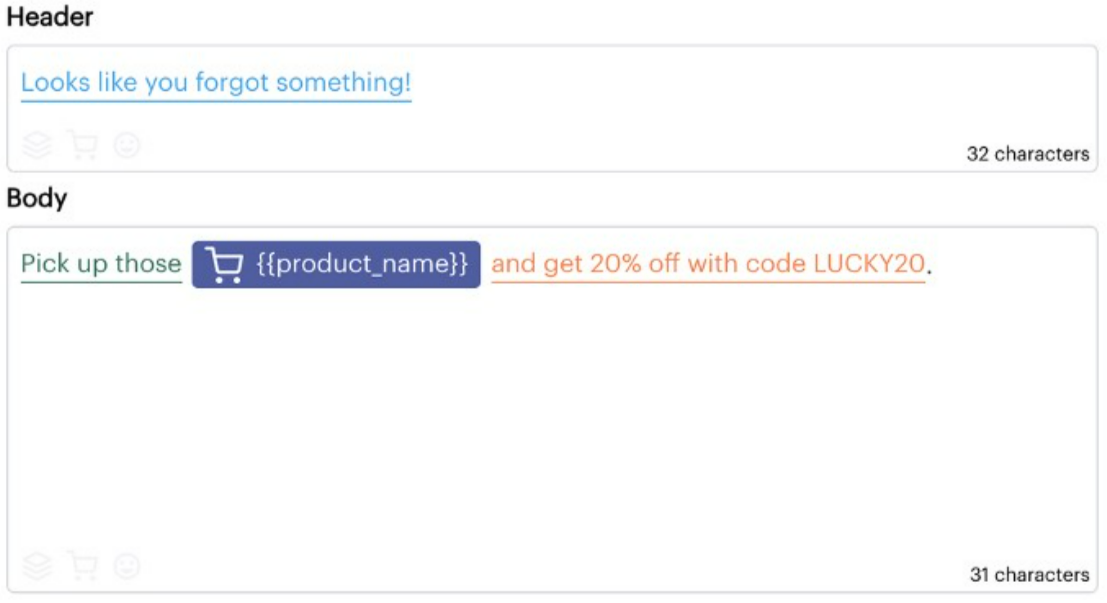
Hit Start Sending!
That’s it!
Once you’ve integrated your catalog, created your template, and hit Start Sending, our recommender logic will take it from there, sending your customers personalized product recommendations that are guaranteed to increase your conversions over your standard/generic marketing messaging.
So, don’t restrict your product recommendations to your app or product search bar or spend tens of thousands of dollars trying to build your own product recommender.
Start sending personalized product and item recommendations in days with Aampe.